Big Data, Big Opportunity: Is Data Science the Key to Universal Energy Access?
A not-so silent revolution is happening around the world. If you are a citizen from a digitalised economy, you are now a quantified human, as there are certainly companies – from Facebook to Amazon to Google – that collectively know what you have done all day. But in many developing economies, most people in rural areas live “dataless” lives. The revolution has not yet reached them – but that is quickly changing.
For instance, if you are a member of an off-grid household in Sub-Saharan Africa, there is a significant chance that you do not have access to basic services like electricity or a bank account. In this context, a new breed of companies is offering goods and services based on the emerging pay-as-you-go (or PAYGO) model, an Internet of Things-enabled microcredit mechanism. This model is creating massive opportunities. When a company sells a PAYGO solar home system to a rural household, for example, something incredible happens: As a credit check is conducted, granular data about the customer’s economic and social situation are recorded for the first time. As weekly or monthly payments are collected, a credit history is built. This data can now be leveraged to improve access to finance, insurance and other productive and life-enhancing assets.
Investors have flocked to PAYGO energy companies, but challenges remain
Most PAYGO companies started out by leasing domestic solar systems to provide off-grid households with clean and reliable lighting and basic energy-enabled services – something the sector has been doing since the early 2010s. Significant progress has been made over this decade, thanks to progressive investment growth from about 10 million dollars invested into the sector in 2010, to over 500 million dollars invested in 2018 alone.
But even though these growing commitments are rightfully celebrated, it is arguably not enough. As Sustainable Energy for All reminds us, investments in both on and off-grid infrastructures together would need to be twice their current number to achieve Sustainable Development Goal 7’s target of universal energy access by 2030. And Lighting Global and GOGLA have found that 67% of the funds dedicated to the PAYGO sector have been distributed among only four players: This is limiting the impact of energy distribution, while possibly over-concentrating the inherent risks of such enterprises (as the recent news about Mobisol’s restructuring would suggest).
The sector faces the dual challenge of gaining further investment, and convincing funds to spread these pledged amounts more broadly across players and countries. This can only be achieved if smaller local PAYGO companies level-up their game in the field of data collection and analysis. It is only by doing so that such players will be able to address the necessary trust conditions for investment: (1) increasing the level of transparency from ground operations to investors; (2) demonstrating a strong local market understanding that investors often lack; and (3) ensuring execution capabilities and exposing the real factors of profitability in remote areas where off-grid technologies expand.
Data-driven strategies unlock the full potential of a company
Many argue that investors’ high growth expectations have led companies to concentrate their efforts on rapid customer acquisition rather than on the quality of their portfolios (thereby significantly increasing the bad-debt ratio, and customer churn). In this context, several new initiatives, co-led by off-grid companies, show a renewed focus on this portfolio dimension as a growth priority. For example, the FIBR project (piloted by BFA* and Mastercard Foundation) conducted several investigations aimed at understanding the value of data for BrightLife, a social enterprise created by FINCA International. The Uganda-based company offers PAYGO products to the unbanked population, who are not eligible for traditional microfinance. In doing so, the company has developed an ideal position to qualify and de-risk the future deal flow of FINCA Uganda, to which BrightLife refers its good payers for FINCA’s microfinance offering – thus constructing an entire upselling strategy based on data valuation. This example, among many others, shows how data science can pragmatically help a company build a relevant competitive advantage to gain profitability and attract investor attention.
Demystifying data science: expectations, reality and ways forward
A sustainable PAYGO business model revolves around a bet on a prospective customer’s future ability to repay his or her loan in full, based on data. Several companies in the PAYGO sector have tried to implement machine learning algorithms to carry out customer segmentation analysis, to predict customer churn, or more frequently, to build credit scores. But more often than not, those predictive models turned out not to be reliable enough to be built into operations. There are likely some very simple reasons why this data analysis didn’t perform well: The companies didn’t know what to expect from data analysis in the first place – or they expected data to behave as a modern-day oracle, in spite of patchy, biased and/or ill-collected data that wasn’t suitable for machine learning algorithms.
In most practical business cases, the simpler the statistical models, the better the chances they’ll be put to good use. Let’s take a common PAYGO problem, for instance: figuring out how to ensure that a customer is repaying his or her lease in a timely fashion. In a recent column, CGAP broke down risk management processes in the PAYGO sector into four main categories: screening, segmentation, customer interaction and appropriate escalation. To properly mitigate credit risk in PAYGO, in most cases, data analysis is merely the cherry on top of a large cake made of efficient operational processes, practical management methods, well-tweaked incentives and general common sense. Customer interaction and appropriate escalation call for iterative problem solving and an experimental mindset, in which sophisticated data analysis plays a small role at best. But screening and segmentation are tough problems to solve, and it turns out that statistical methods are custom-made for efficiently describing any complex, multi-dimensional sample of prospects or customers.
To screen customers at the point of sale, a predictive model can be built as an additional component to a global credit score. (Other components could be ratings from a credit bureau, or qualitative character data derived from small-talk with the village chief.) Granularity is key when it comes to building rich and insightful credit scoring models. Often, predictive models built only with data collected prior to the point of sale tend not to be predictive enough to be reliably embedded into operations. Of course, as one feeds data collected after the point of sale (e.g.: repayment history and aftersales interactions) into the model, the predictions’ quality improves significantly.
But since a company needs to foresee the future, optimization problems can arise. For instance, how soon in the timespan of the company-customer relationship can we reliably predict how things are going to end? Are there any data parameters that vary only slightly, that are not considered relevant in the analysis, but that are going to make a huge difference in the end? Which unthought-of aspects or characteristics turn out to be material (i.e. significantly increasing predicting power) in our predictive model? The answer varies between contexts. But when data is well-structured and has been consistently collected, building a basic yet efficient predictive model can be done in a couple of hours.
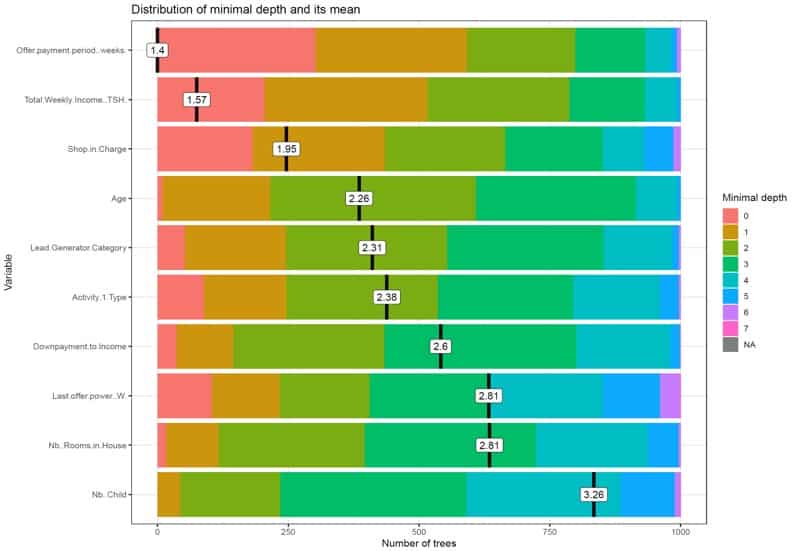
Distribution of minimal depth and mean of generic variables used in the fitting of a random forest
This example of a churn predictive model is based on a machine learning algorithm for a set of customers in Tanzania. Through it, we are able to determine with 70% accuracy which customers are likely to pay on time and which are likely to churn, using only data collected at the point of sale. However this is not enough, since adding early-stage interaction data to the model increases its predictive accuracy to 80%, and reduces the false positive error rate to 30%, thus meeting the expected quality of an embedded credit risk indicator.
“If you can’t measure it, you can’t improve it”
The above quote from management guru Peter Drucker holds true for off-grid energy. Many initiatives are already providing the entire off-grid industry with stronger data science capabilities, helping them to maximize their impact on the ground, while increasing their attractiveness to investors. If the industry were to put data at the heart of its expansion strategies with a stronger focus on data granularity in PAYGO, off-grid companies would access further levers of growth and reach greater operational efficiency – and this could trigger the extra investment dollars needed to bring economic justice to the bottom of the pyramid.
That being said, the path towards models like PAYGO that involve banking the unbanked needs to be transparent and publicly debated. Faulty assumptions, biased credit risk models or risky business practices could wrongly screen out prospects as potential credit risks, denying both further electrification prospects and access to credit to large swathes of customers across rural Africa. But if the sector can navigate these risks, the rewards of a data-centric approach could be transformative.
Guilhem Dupuy is Investment Director of the GAIA Impact Fund.
Thibault Lesueur is Chief Marketing Officer and Co-Founder of Solaris Offgrid.
Photo courtesy of Russell Watkins / DFID.
- Categories
- Energy, Technology